n the ever-evolving era of artificial intelligence, OpenAI’s Generative Pre-trained Transformers (GPT) have revolutionized how machines approach language. The introduction of GPT-4 was a giant step forward from its counterpart, GPT-3.5. Even though the two models are extremely effective language-processing tools, some enhancements in GPT-4 have revamped its use in natural language processing (NLP) and multitask capabilities.
A Brief Guide to Construction Jobs
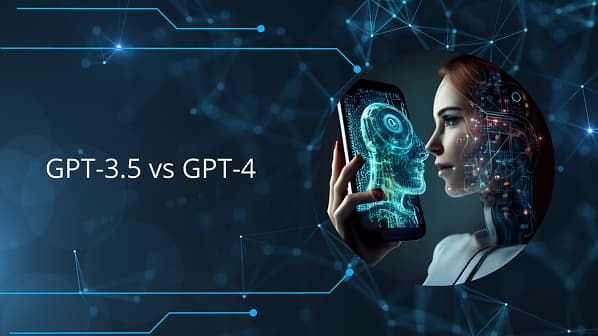
1. Model Architecture Technical Developments
GPT-4 introduces a big jump in model complexity from GPT-3.5, with an extended and more powerful neural network design. The approximately 175 billion parameters used by GPT-3.5 are significantly surpassed by GPT-4 because it utilizes optimized techniques to augment its depth alongside contextual processing abilities. The enhanced upgrades of GPT-4 enable better data processing thus making it better suited for intricate reasoning operations and complex question responses compared to the original GPT-3.5 design.
The primary difference between GPT-4 involves its ability to process longer texts because it supports a token limit stretching up to 32,768 values equivalent to 50 pages of written content. GPT-3.5 shows limitations during tasks requiring analysis of lengthy texts and the production of extensive written content because its maximum token count stands at 4,096. This increased input length enables GPT-4 to handle advanced multi-page documents without compromising contextual specificity, and thus it is the superior choice for long-content generation and document complexity.
2. Multimodal Features
Whereas GPT-3.5 can only work with text-based data, GPT-4 introduces groundbreaking multimodal features. It can handle text and image inputs, significantly expanding its use cases. For applications such as law, medicine, and marketing, multimodality allows GPT-4 to analyze diagrams, charts, or visual aids in addition to text-based material for deeper comprehension and outputs. For instance, GPT-4 has demonstrated high performance in clinical application, decomposing text and graph information in reports to support diagnosis recommendations. Multimodality thus gives GPT-4 a distinct advantage in building domains that demand hybrid data processing.
3. Contextual Control and Accuracy of Response
GPT-4 is more context-specific and nuanced questioning. It has 19–29% fewer hallucinations, or mistakes, and thus is a more reliable tool in complex decision-making environments. Its additional contextual knowledge shows up as well in its capacity to sustain coherence in lengthy, multi-turn conversation, where GPT-3.5 often is not able to continue accurately beyond a few turns.
Furthermore, GPT-4 is more superior when it comes to creative output, problem-solving, and rational thought. As an example, under healthcare or jurisprudence conditions, GPT-4 executes better on knowledge-based tasks, i.e., diagnostic analyses or case outcome prediction. Under the task of a clinical trial outcome prediction, GPT-4 had a 92% rate against the 87% for GPT-3.5. The clear difference reveals the sophisticated methods that GPT-4 follows while processing data.
4. Training Data and Knowledge Scope
The training data used by GPT-4 includes more recent content than the data used by GPT-3.5. GPT-3.5 retained its training data up until 2021 but GPT-4 operates with current content leading to responses with modern knowledge perspectives. GPT-4 delivers superior domain-specific answers because it processes recent updated knowledge from its broad training sources about new technologies and industry trends.
The extensive training scale of GPT-4 enhances its performance in handling cross-language situations and general knowledge applications. It is more accurate at translating and even provides culturally responsive content, improving its applicability in non-English-speaking nations. GPT-3.5, on the other hand, is adequate in several languages but lacks both fluency and grammatical prowess in less-spoken languages.
5. Computational Requirements and Cost-Efficiency
Despite its improvements, GPT-4 remains more computationally intensive than GPT-3.5. Its larger size and multimodality are computational expensive to launch, frequently translated into higher launching costs. Where resources are in short supply and for smaller-sized organizations or use cases, the GPT-3.5 remains a suitable and low-cost alternative.
However, computational cost trade-offs are well worth it for companies requiring accuracy, scalability, and high-level functionality. For use cases such as writing long documents, processing complex queries, or computing multi-source datasets, the computational investment in GPT-4 is worthwhile.
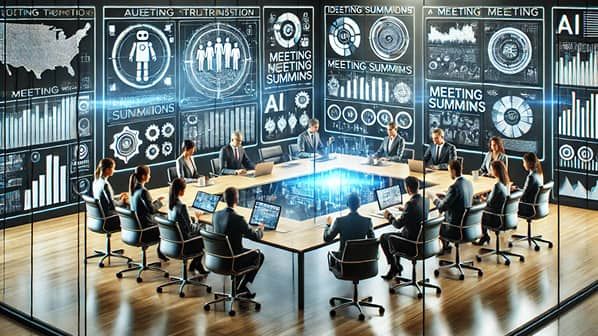
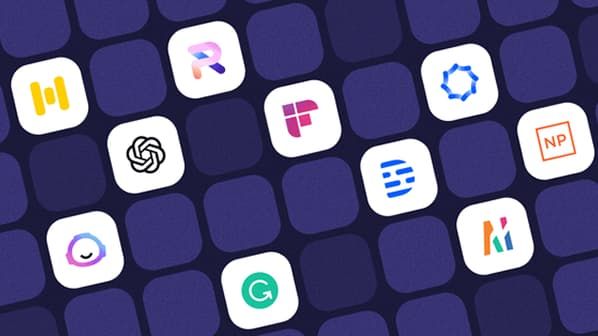
Guess you like
-
Why You Need to Try Interactive Movie Adventure Games
-
A Definitive Guide to the Best Classic Sports Cars
-
Uncover the New Jeep Lineup 2025 and Its Attributes
-
Down-to-Earth Guide to Linking Your Xbox One Controller with Apple Gadgets
-
Major SEO Ranking Tools for Effective Website Optimization in 2025
-
Practical AI-Driven Strategies to Increase Revenue Growth
Trending
-
1
The Most Useful Gadgets Spicing Up Every Beach Lover’s Experience This Summer
-
2
Starter’s Guide to Buying the Right Camera for Beginners
-
3
How Non-Experts Can Easily Enhance Website Load Speed While Browsing
-
4
Things You Should Never Leave in The Car on Hot Days
-
5
6 Accessories to Deliver the Best Gaming Experience
-
6
5 Artificial Intelligence Features Already Making Your iPhone Smarter